Fiona Maclean1
1 Douglass Hanley Moir, Franklin.ai, Sydney, NSW, Australia
Abstract for USCAP 2025, Boston, United States
Background
We have previously shown that our Prostate AI model performed strongly in classifying 45 findings in prostate core biopsy (PCB) and transurethral resection of prostate (TURP) specimens.
Subsequently we evaluated performance in quantifying tumour extent and calculating Gleason Pattern (GP) proportions. Additionally, we measured the precision and generalisibility of our model across classification tasks under varying operational conditions.
Design
The model was developed using >70,000 PCB and TURP digitised whole slide images (WSI) and performance was tested on >1,700 WSIs for quantification tasks. Cancer involvement and the proportion of GPs within tumour on WSIs was evaluated by measuring bias between the AI model and unassisted pathologists by assessing the strength of agreement (correlation) in outputs.
Model precision (repeatability) was assessed by measuring the variability in classification performance (Area under the Curve, AUC) on a test set of 148 slides scanned 3 times using the same scanner by the same operator.
Generalisability (reproducibility) was determined by measuring the variability of model performance when a 145 slide test set was scanned once on three different scanners by three different operators. These studies were performed on two different scanner types, Leica 450DX and Philips UFS300. 1136 images with malignancy were included in the quantification test set and 71 images with malignancy in the precision test set.
Results
The mean bias between the model and pathologists in % cancer involvement and GP proportions was <5% and <1% respectively and was statistically acceptable (predefined threshold of ±5%).
On average, the model predicted 0.27, 0.71 and 0.96% higher proportions of GP3, GP4 and GP5 respectively. Strong positive correlations between model predictions and human measurements were observed across all GP proportions (r≥0.75). Repeatability showed within-scanner AUC variability of < 5% for Leica (range: -0.32 to 0.11%) and Philips (range: -0.75 to 0.53%).
For reproducibility, statistical agreement was demonstrated between-scanner and between-operators for both Leica (range: -0.32 to 0.11%) and Philips (range: -0.32 to 0.75%) scanners.
Conclusion
Cancer involvement and GP proportions predicted by the AI model were highly correlated to measurements produced by that of pathologists. The AI model demonstrated high precision and generalisability in the classification of prostate clinical findings across variable settings.
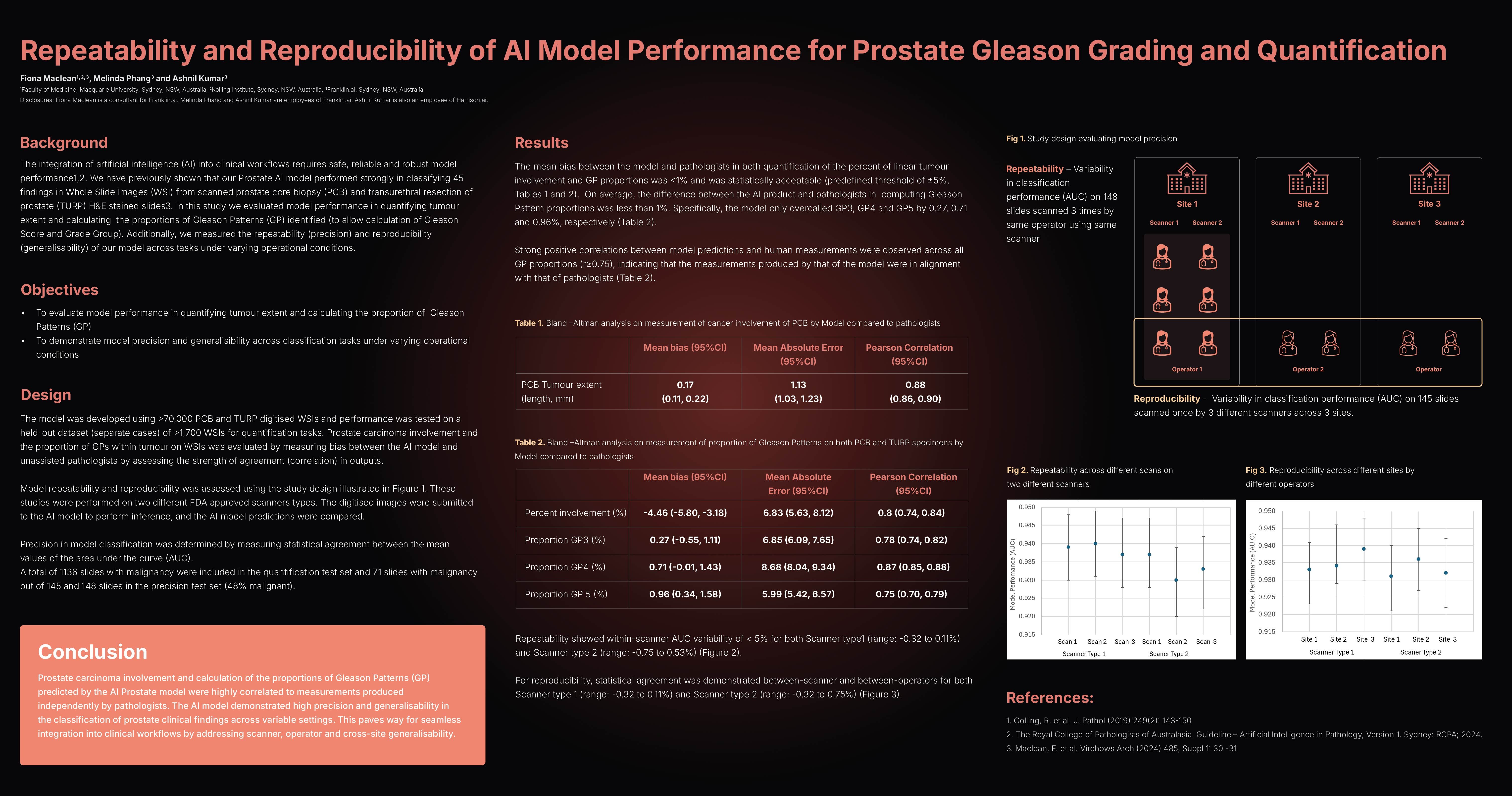
Click here for full resolution poster